Although the number of articles published on news portal sites has been increasing in recent years, there is a growing demand for appropriate news recommendations to improve the user experience. In this paper, we focus on user’s personality traits and propose a method to estimate personality traits only from news browsing logs collected from actual services in order to realize a news article recommendation system that takes user’s personality traits into account.
Since news browsing logs contain article IDs that identify articles, the data becomes sparse and high-dimensional when the IDs are expanded horizontally at the data processing stage. To solve this problem, we calculated low-dimensional embedded features using a natural language processing technique that regards users as documents and viewed article IDs as words. In addition, we extracted features that take into account the user context by aggregating the browsing time and browsing rate of articles for each user and performing multiple statistical processes.
We constructed a supervised machine learning model using these features as explanatory variables and personality characteristics collected from a crowdsourcing questionnaire as objective variables. We evaluated the accuracy of the estimation of personality characteristics using multiple machine learning algorithms.
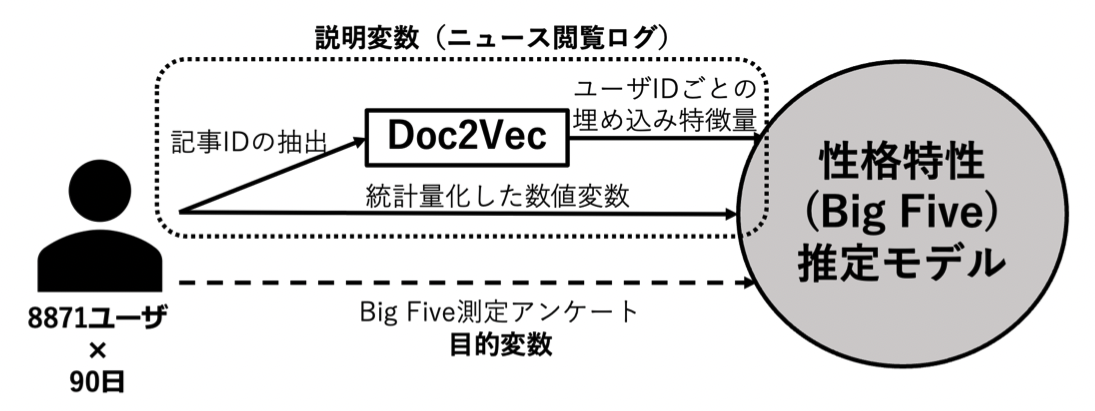