Focusing on the emotional state which is closely related to the rationality states, we constructed machine learning models to estimate the emotional state (mood score) from the user’s search keywords by combining (1) values obtained from various sensors on smartphones, (2) self-reported subjective data obtained through the Experience Sampling Method (ESM), and (3) search keywords in a search engine. By combining these three types of data, we constructed a machine learning model to estimate the emotional state (mood score) from the user’s search keywords. These data were collected through a large-scale experiment involving 400 users over a period of about 90 days. Our system was deployed in the commercial stack, and a large-scale data analysis with more than 11 million users was conducted.
Interestingly, our proposed “Nation-wide Mood Score,” which bundles the mood values of users across the country, (1) shows the daily and weekly rhythm of people’s moods, (2) explains the ups and downs of people’s moods in the COVID-19 pandemic, which is inversely synchronized to the number of new COVID-19 cases, and (3) detects the linkage with big news, which may affect many users’ mood states simultaneously, even in a fine-grained time resolution, such as the order of hours. Furthermore, (4) we confirmed the existence of certain class of web advertisement whose user reactions are influenced by their mood.
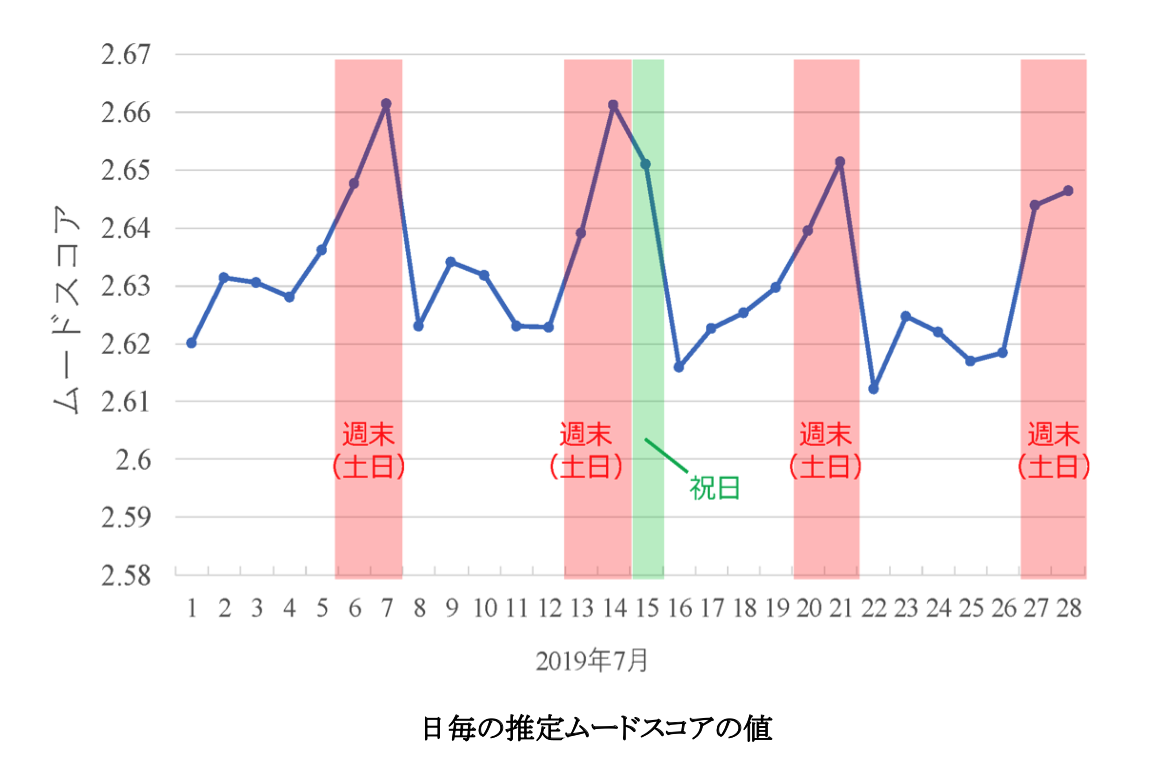
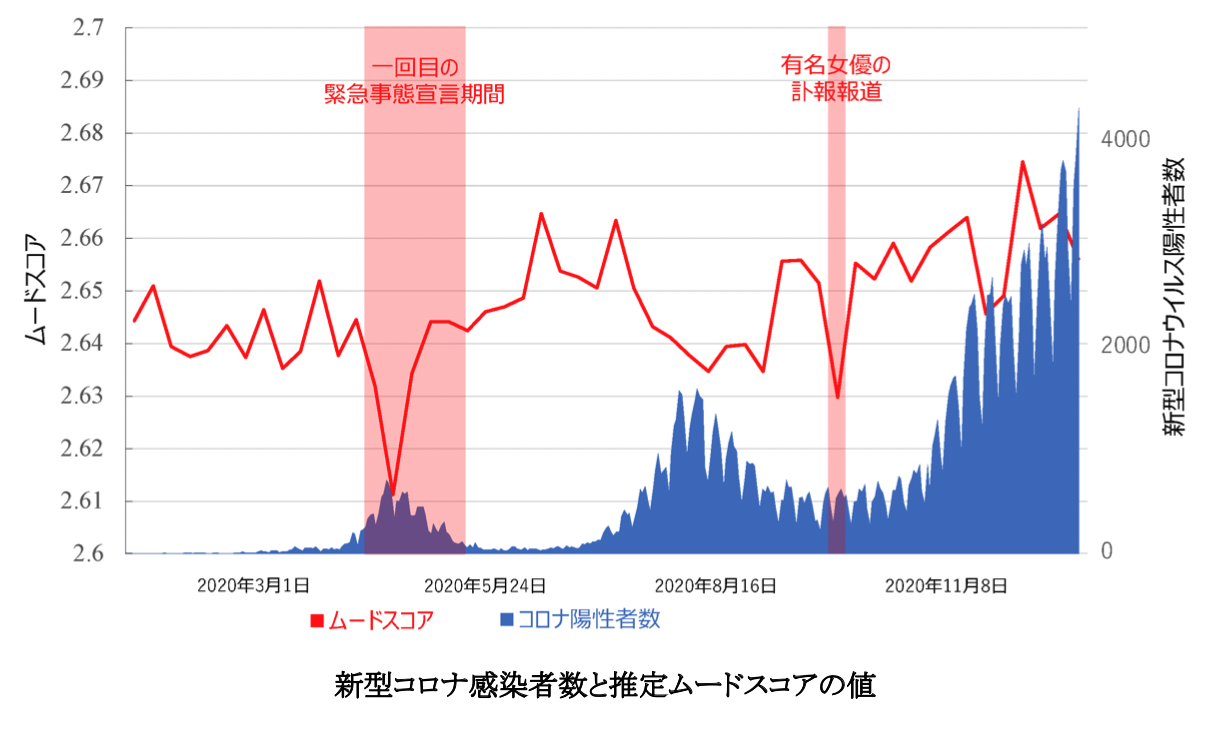
研究成果
- Sasaki, W., Kawase, H., Miyahara, S., Tsubouchi, K., & Okoshi, T. (2021). Nation-wide Mood: Large-scale Estimation of People’s Mood from Web Search Query and Mobile Sensor Data. arXiv preprint arXiv:2111.05537. https://doi.org/10.48550/arXiv.2111.05537